NEURAL NETWORK SHIPPING MODEL
By Alfonso Llanes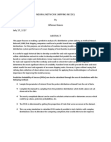
This paper focuses on making a predictive analysis of a distribution system utilizing an Artificial Neural Network (ANN) that shipping companies could use to predict transit time over a complex scattering of destinations. For this purpose, an introduction of machine-learning models capable of predicting distribution system performance of ocean shipping of merchandise is presented. It is useful to apply historical data to develop a model for each sub-segment of a Transportation route within a distribution network in order to develop models that predict the ocean transit sub segments based on various origins and destinations. Linear regression, tree and random forest model can be used for each sub-segment to find the weekday and month in which the trade transporter segment determines the most significant drivers of variability. Random forest models provide the best and most robust results for most sub segments of an ocean shipping route. However, it goes without saying that refining data collection of observations more accurately for applying these methodologies is of outmost importance for improving the model accuracy. Predictive Probability of Success (PPOs) can also be calculated through the use of simulations with the following method: ♦ Sample the parameter of interest from the poster distribution attained from the set of data. ♦ Complete the dataset by sampling from the predictive distribution which holds values not yet observed in the data. ♦ The newly completed dataset can be used to calculate criteria used to determine success which could be p-values, posterior probabilities, etc. ♦ The PPOS is determined by getting the proportion of trials that were successes in the dataset. ♦ This way using simulation to calculate PPOS makes it possible to test statistics with complex distributions since it alleviates the computing complexity that would otherwise be required.